New Method for Clustering Data Generated from Linear Subspaces
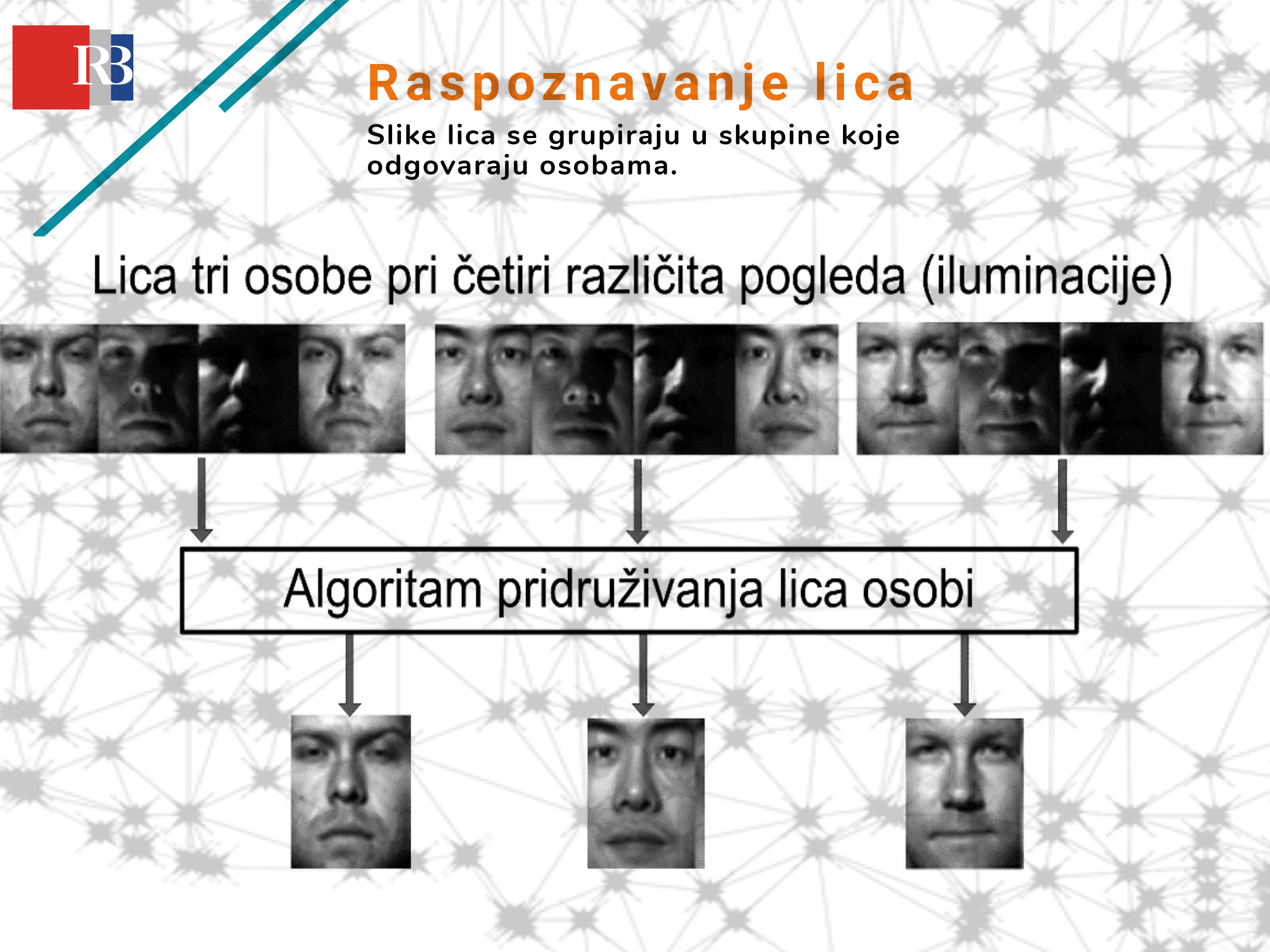
Researchers form the RBI Laboratory for Machine Learning and Knowledge Representation Maria Brbić and Ivica Kopriva have developed a new method for data clustering based on the linear subspace model as the generator of the corresponding functional groups. These findings were published in one of the most influential scientific journals in the field - 'IEEE Transactions on Cybernetics' (IF 8.803).
Machine learning is a branch of artificial intelligence that studies computer algorithms for automatic data processing. In other words, this is the process of discovering knowledge from a large amount of data, whereby computer systems automatically improve their processes through experience. Machine learning is the foundation of today's data science, and it can be divided into categories according to the amount of prior knowledge required for learning task, i.e. supervised, unsupervised and semi-supervised machine learning.
One of the fundamental problems in computer science within a category known as unsupervised learning is data clustering. Namely, in contrast to the supervised machine learning, where we provide the input and target data to train the algorithm, and in the end we pick the function that best describes the input data, in the unsupervised machine learning process we expect from algorithms to accomplish the learning task using only information derived from the data itself (data driven methods). Simply put, there is no teacher during an unsupervised learning process, there is no one to provide the exact knowledge, and data are given without a target value. In this case, the goal is to detect structural pattern in the data, or to discover what data mean to be able to describe it better to the users.
Machine learning is one of the most exciting areas of computer science due to the many application possibilities such as pattern recognition and in-depth analysis of data, robotics, computer vision, bioinformatics, computer linguistics, all the way to innovative applications in medicine.
Some of the most common applications of data clustering in medicine relate to image segmentation, such as in CT images (clusters are organs), PET images (clusters are tissues), microscopic images of histopathological preparations (clusters of tissues and / or cells), optical coherence tomography images (e.g. clusters are layers within the retina).
"The applications that we have illustrated in this new paper relate to the identification of faces or grouping of faces of individuals into clusters that suit people, then the recognition of speakers, i.e. the grouping of speech features into clusters that suit people, and handwriting the written numbers, or grouping images into clusters which correspond to digits from 0 to 9. "- explained Ivica Kopriva.
The developed methods of data clustering in the above examples are based on the model according to which the data are generated from the corresponding linear subspace within each group.
Based on this model, researchers have developed algorithms that give very competitive results on the clustering of demanding data sets.
"The key element in this approach is the method for learning representation matrix from data. The good representation matrix has to be sparse with a low rank. Instead of standard convex measures of rank and sparsity, the functions we have proposed in this paper stand for more accurate measures of rank and sparisty . "- explains Maria Brbić.
The resulting nonconvex optimization problems were solved using an alternating direction method of multipliers with established convergence conditions of both algorithms. Results on synthetic and four real-world datasets show the effectiveness of developed GMC-LRSSC and S₀/ℓ₀-LRSSC methods in comparison with the state-of-the-art methods.
"The new algorithms have significantly improved accuracy compared to the existing methods on all tested applications," - concludes Brbić.